Introduction
Logistics is crucial for the global economy. Businesses must stay updated with trends, and customer demands to stay ahead. Data science helps organizations make informed decisions by analyzing data. It optimizes supply chains, improves logistics performance, and offers personalized solutions. Data science allows industries to adapt to market changes and stay competitive.
Data science is a powerful tool in the logistics industry, enabling businesses to analyze vast amounts of data and make informed decisions. This abundance of data provides significant opportunities for companies to remain competitive.
Industries Overcoming Logistics Challenges Using Data Science
Global supply chain companies need help using the vast amount of data they collect for decision-making and risk management. According to a study by McKinsey, around 1.2 trillion gigabytes of data are created annually. These challenges include dealing with data spread across multiple applications, identifying patterns, detecting deviations, estimating probabilities, illustrating relationships, and categorizing data points. It is also difficult to make predictions because logistics data depends heavily on many internal and external applications and is influenced by unpredictable weather and geopolitics.
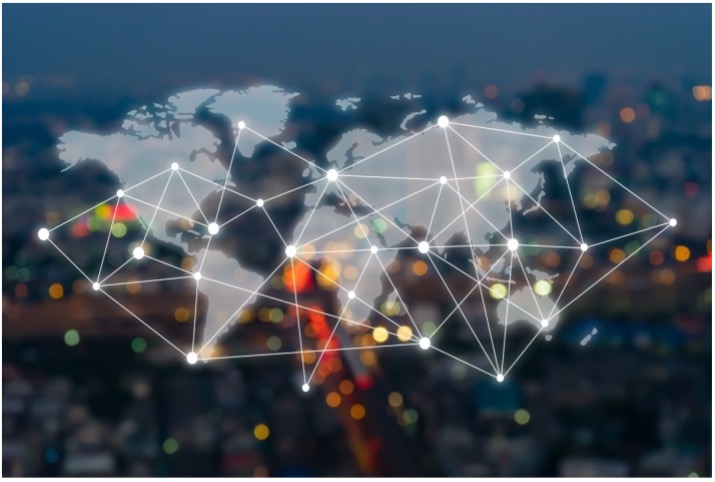
A study by DHL found that businesses that incorporate data analytics in their supply chain operations can gain up to 19% higher profitability than their counterparts. By utilizing the expertise of data scientists, industries can make informed decisions that drive growth and efficiency. The analysis of historical data provides valuable insights that can help industries forecast demand accurately, prevent stockouts, and reduce excess inventory. Capgemini’s report highlights the importance of data analytics in reducing forecast errors by up to 50%, which presents significant potential for streamlining business operations and enhancing profitability.
Data insights can significantly improve logistics efficiency by analyzing traffic patterns, weather conditions, and delivery time windows. This analysis helps optimize delivery routes, reduce transportation costs, and ensure on-time deliveries. For example, UPS’s ORION system, which uses data science algorithms to optimize delivery routes, saved 10 million gallons of fuel and reduced CO2 emissions by 100,000 metric tons in just one year. Analyzing customer data can help industries optimize logistics, offer personalized experiences, and increase customer loyalty. Accenture found that personalized logistics services can increase customer retention by up to 30%.
Case Studies
Furthermore, these case studies demonstrate the tangible benefits of data science to staying current with logistics trends and meeting customers’ demands.
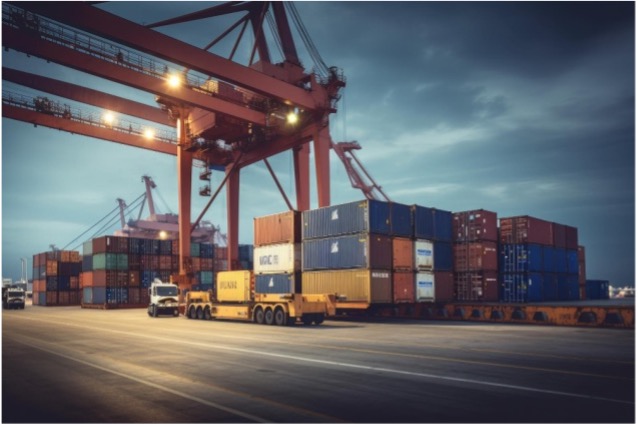
- UPS is now implementing data logistics with ORION, showing that data-driven route optimization reduced the distance traveled by their drivers by 100 million miles, resulting in significant cost savings and reduced carbon emissions.
- DHL implemented real-time tracking and monitoring to improve supply chain visibility by up to 70%, improving operational efficiency and customer satisfaction.
- A study by Gartner found that companies that leverage data science in warehouse management can achieve up to 30% improvement in inventory accuracy and a 20% reduction in carrying costs. Deloitte implemented predictive maintenance toreduce maintenance planning time by 25% and reduced overall maintenance costs by 10%.
- A study by Accenture found that companies that leverage data science for risk management can reduce supply chain disruptions by up to 40%, resulting in improved resilience and customer satisfaction. A study by McKinsey found that companies that leverage data science in logistics can achieve up to a 20% reduction in transportation costs and a 15% reduction in inventory carrying costs.
- The US Department of Energy found that optimizing transportation routes using data science techniques can reduce carbon emissions, contributing to environmental sustainability.
Oracle Transportation Management and Data Science
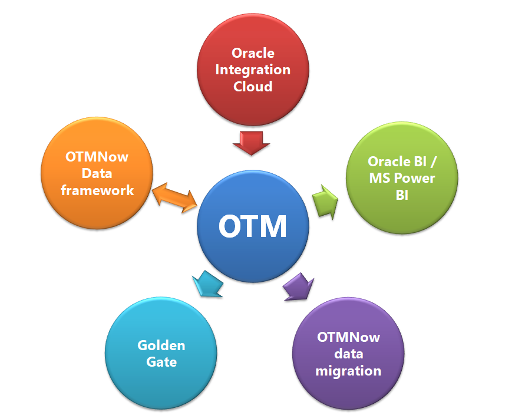
Oracle Transportation Management (OTM) is a global leader in logistics application that helps companies manage their logistics activities across their supply chains. With OTM, businesses can reduce costs, optimize service levels, and automate their business processes globally. OTM is built on a robust event-based architecture and complex workflow framework, making it an excellent tool for data exploration and modeling. OTM encompasses various built-in modules, including logistics network modeling, machine learning, and intelligence, which help model and visualize data. Additionally, OTM integrates with various applications using different protocols to collect data.
KSAP Technologies has been a leader in assisting customers with implementing OTM and achieving their logistics objectives for the past 25 years. The proprietary OTMNow™ framework integrates seamlessly with OTM and Oracle cloud architecture to create an ecosystem with all the required tools to meet enterprise needs and implement data science solutions.
OTMNow™ Data framework is designed with an in-depth understanding of OTM to provide customized and high-quality solutions. It offers flexibility and scalability to adapt to ever-changing business needs. The framework integrates OTMNow migrate and data maintenance to help customers with data collection, cleaning and exploration. Having a clean and complete data is critical for the successful data analysis and insights. The framework provides various templates that can help customer to build and ingest the data from various sources. The framework implement various neural netowork and linear regression models to relate the data and fill the data gaps to make the data meaningful. The cleansed data then integrated into OTM data tables for further processing through OTM workflows.
KSAP’s expertise and OTMNow product suite that align with Data Science solutions
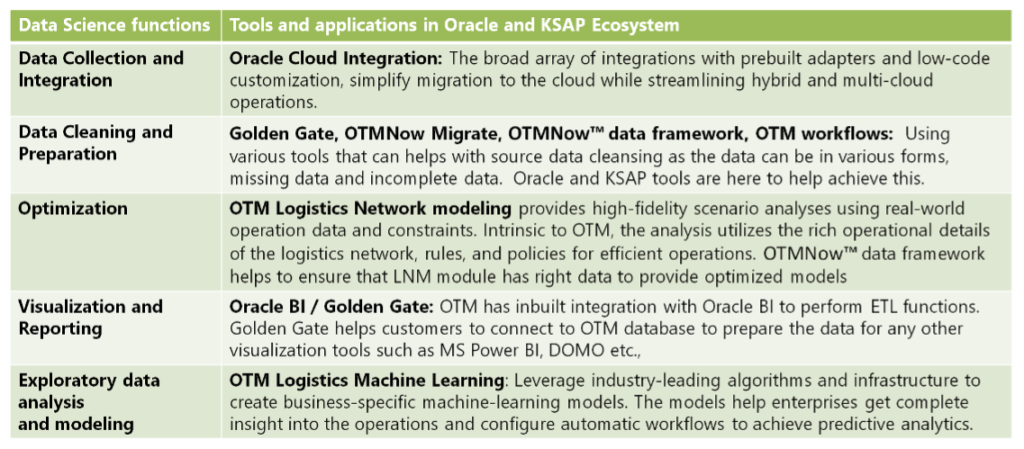
Key benefits of Data science throughout logistics life cycle
Discover the numerous benefits of data science in logistics with our comprehensive analysis and supporting data. Explore how data science can enhance your logistics operations and improve your bottom line. Don’t miss out on this valuable opportunity to optimize your logistics strategy.
- Improved demand forecasting: Data science techniques such as time series analysis and machine learning algorithms analyze historical sales, market trends, and external factors to forecast demand accurately, resulting in improved inventory management and reduced costs.
- Enhanced route optimization: Leveraging network modeling and optimization models, companies can identify the most efficient routes that minimize travel time, fuel consumption, and transportation costs. A case study by UPS showed that implementing data-driven route optimization reduced the distance traveled by their drivers by 100 million miles, resulting in significant cost savings and reduced carbon emissions.
- Real-time tracking and monitoring: Data analytics and visualization allow for real-time tracking and monitoring of shipments with visibility into their current location, condition, and status. Integrating data from various IoT devices like GPS and sensors, companies can proactively identify potential delays, suggest alternative routes to mitigate risks, and provide accurate delivery updates to customers.
- Efficient warehouse management: Predictive analytics help companies optimize operations by accurately predicting demand, which maximizes inventory replenishment, reduces stockouts, and improves order fulfillment rates.
- Predictive maintenance: By analyzing data from sensors and equipment monitoring systems to proactively detect patterns and anomalies in the data, predict equipment failures, and schedule maintenance activities, thus preventing costly breakdowns, minimizing downtime, and extending the lifespan of assets.
- Risk management: Techniques like random forest classifiers help companies develop contingency plans and optimize their supply chain to minimize disruptions by reducing data noise and outliers.
- Sustainability: Linear regression models help you analyze factors causing delays, wastage, and logistics inefficiencies. Companies can implement sustainable logistics practices by analyzing data on fuel efficiency, vehicle utilization, and alternative energy sources.
Future Trends and Opportunities
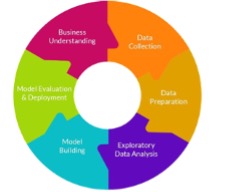
Data science is rapidly evolving and shaping the logistics industry. Emerging trends present exciting opportunities to optimize operations and improve decision-making. Integrating various cutting-edge technologies in AI, machine learning, big data analytics, IoT, and data visualization helps transform enterprise data into actionable insights.
To implement these trends in transportation management, organizations need to follow a systematic approach:
Data Collection and Integration: Establish a robust data collection infrastructure and integrate data into a centralized repository for further analysis.
Data Cleaning and Preparation: Ensure data quality by cleaning and preprocessing methods like removing duplicates, addressing missing values, and standardizing formats.
Advanced Analytics and Modeling: Apply AI and ML techniques to analyze the collected data and build predictive models.
Visualization and Reporting: Develop interactive dashboards and visualizations to present insights in a user-friendly manner. These tools should provide real-time updates, customizable views, and drill-down capabilities for deeper analysis.
Continuous Improvement: Regularly monitor and evaluate the implemented solutions to identify areas for improvement. Incorporate feedback from users and stakeholders to refine the models and enhance decision-making processes.
With KSAP’s logistics expertise, innovative solutions and OTMNow™ product suite, organizations can achieve significant benefits such as cost savings, improved efficiency, enhanced customer satisfaction, and sustainability by embracing current trends and utilizing data science techniques. Effective utilization of data science techniques can provide a competitive edge to enterprises in this ever-changing world.
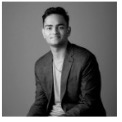
Author: Sathwik has worked in the logistics industry for the last three years, specializing in research on trends and cutting-edge technologies. Sathwik is a computer science graduate from Georgia Tech, focusing on intelligence and information management.
Key Contributor: Raghu Lakshman: Raghu has over 25 years of experience with a focus on Supply Chain and Logistics. As a Sr. Solution Architect, Raghu has been helping customers across the globe with their supply chain initiatives.